LLMs - Large Language Models
LLMs are neural networks that can process and generate natural language text.
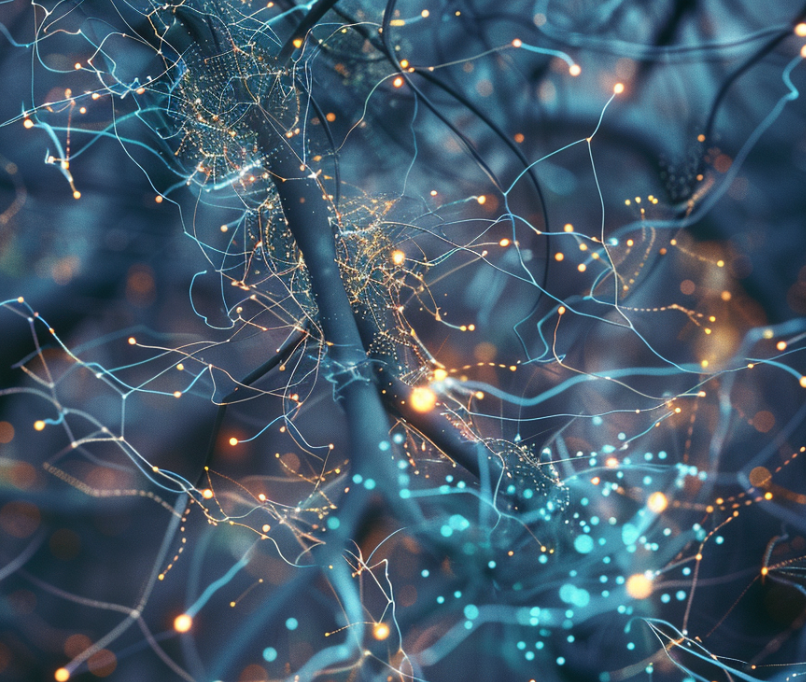
TRAINING PHASE
They are trained on a dataset of billions of sentences using unsupervised learning techniques. In the training process LLMs learn what is the most likely word to came next to the previous one based on huge amount of data.
INPUT BY USER
LLMs accept as input a text prompt by a user and in relation with it generate in output text, word by word (token by token).
GENERATION OF THE OUTPUT
The generation process consists in predicting the next word on the base of previously generated words. LLMs are trained in doing this without any consciousness which is a prerogative of the human mind.
Building a Simple Large Language Model
In this example we use as data the dystopian novel “Nineteen Eighty-Four – 1984” by English writer George Orwell, published on 1949.
Using the text of the novel as a data source, the following tables were produced. I show only a part of them:
Word/Token | Occurrences |
the | 6249 |
of | 3309 |
a | 2482 |
and | 2326 |
to | 2236 |
was | 2213 |
He | 1959 |
It | 1864 |
in | 1759 |
that | 1457 |
had | 1311 |
his | 1079 |
you | 1011 |
not | 827 |
with | 771 |
as | 672 |
At | 654 |
they | 642 |
for | 615 |
IS | 614 |
but | 611 |
be | 608 |
on | 604 |
were | 583 |
there | 559 |
Winston | 526 |
him | 512 |
i | 495 |
which | 443 |
s | 439 |
one | 426 |
or | 424 |
… | … |
Word/Token | Word Next | Score | Probability |
of | the | 743 | 0,01139 |
It | was | 589 | 0,00903 |
in | the | 574 | 0,00880 |
He | had | 355 | 0,00544 |
he | was | 273 | 0,00418 |
on | the | 230 | 0,00352 |
was | a | 225 | 0,00345 |
there | was | 223 | 0,00342 |
to | the | 212 | 0,00325 |
O | Brien | 205 | 0,00314 |
to | be | 203 | 0,00311 |
and | the | 203 | 0,00311 |
had | been | 202 | 0,00310 |
the | party | 195 | 0,00299 |
at | the | 183 | 0,00280 |
that | he | 167 | 0,00256 |
from | the | 161 | 0,00247 |
with | a | 158 | 0,00242 |
did | not | 148 | 0,00227 |
that | the | 147 | 0,00225 |
of | a | 145 | 0,00222 |
of | his | 145 | 0,00222 |
out | of | 142 | 0,00218 |
was | not | 130 | 0,00199 |
with | the | 127 | 0,00195 |
he | could | 124 | 0,00190 |
it | is | 124 | 0,00190 |
in | his | 123 | 0,00188 |
in | a | 122 | 0,00187 |
They | were | 122 | 0,00187 |
seemed | to | 115 | 0,00176 |
was | the | 110 | 0,00169 |
could | not | 109 | 0,00167 |
he | said | 109 | 0,00167 |
the | same | 103 | 0,00158 |
for | the | 101 | 0,00155 |
by | the | 95 | 0,00146 |
for | a | 92 | 0,00141 |
into | the | 92 | 0,00141 |
she | had | 87 | 0,00133 |
as | though | 82 | 0,00126 |
they | had | 80 | 0,00123 |
that | it | 80 | 0,00123 |
have | been | 79 | 0,00121 |
and | a | 78 | 0,00120 |
it | had | 77 | 0,00118 |
The | other | 76 | 0,00116 |
of | them | 76 | 0,00116 |
to | him | 75 | 0,00115 |
the | telescreen | 75 | 0,00115 |
BIG | BROTHER | 73 | 0,00112 |
… | … | … | … |
This is a simple diagram to understand how the text is generated word by word.
For example, if I start with BIG, LLM will probably generate BROTHER, and continuing we can produce this sentence:
BIG | BROTHER | was | a | sort | of | the | thought | … |
Probability | 0,00112 | 0,0005 | 0,00345 | 0,00095 | 0,00104 | 0,00023 | 0,00087 |
ChatGPT is a LLM
By using “prompt” mechanism you can ask ChatGPT for what you want using the natural language.
But how ChatGPT “UNDERSTAND” text inserted by the user?
The text is transformed and each word represented by a code that computer can processed.
A way to represent individual words is Word2Vec technique in natural language processing (NLP), in which each word is represented by a vector (a set of numbers). This helped a computer to assign a meaning to the word.
Word2Vec stands for “words as vectors”. It means expressing each word in your text corpus in n-dimensional space. The word’s weight in each dimension defines it for the model.
The meaning of the words is based on the context defined by its neighboring words where they are associated.
A simple example of word representation using the Word2Vec approach in two-dimensional space.
Man = [1,4]
Woman = [1,3]
Manager = [4,2]
Actress = [4,1]
Manager | - | Man | + | Woman | = | Actress |
[4,2] | [1,4] | [1,3] | [4,1] |
In the following picture we have the graphic representation.
This is what happens when you sent some prompt to ChatGPT.
- The text is converted and split in tokens;
[10,10], [10,31], [10,15], [14,44], [8,5], …
(you, are , an, ICT, specialist, with, a, lot, of, experience)
- An algorithm (like ChatGPT) makes some prediction and output text word by word.
[10,10],…
(you,can,have, an, important, and, well-paid, job)
ChatGPT and PROMPT ENGINEERING
Let us now analyze some techniques to better exploit the potential of ChatGPT.
DIRECTIONAL PROMPTING
If you submit the same question to ChatGPT many times, you will likely receive different answers.
How can you use directional prompting in order to get more precise answer?
You have to give more information and to be more descriptive when you define a prompt. You have to give clear instruction. This will help the model to understand of what you want. If you ask for generic question, you receive generic answer.
Generic question:
More specific question:
More contextual and specific question:
OUTPUT FORMATTING
If you want to have a specific output or format of the output from ChatGPT, for example CSV (Comma Separated Values), Microsoft Excel, Microsoft Word or simply txt or maybe code as well, you have to specify as in the following examples.
We want statistical data in CSV format:
BIBLIOGRAPHY/WEBOGRAPHY
[01] openai.com;
[02] KENNETH WARD CHURCH, Emerging Trends Word2Vec, IBM 2016;
Not generated by AI tools or platforms.
{[(homo scripsit)]}